- EFIM30051 Data Analytics and Artificial Intelligence for Business UOB Assignment Answer UK
- EFIM20003 International Business Management Assignment Answer UK
- 07 37249 Business Communication Skills B Assignment Answer UK
- 07 37248 Business Communication Skills Assignment Answer UK
- 07 32264 Responsible Business: Theory and Practice Assignment Answer UK
- 08 32101 Business, Economy and Government Assignment Answer UK
- 07 33171 Business Law Assignment Answer UK
- ICAEW Insurance Business Planning Assessment Answer
- ICAEW Banking Business Planning Assessment Answer
- ICAEW Taxation Business Planning Assessment Answer
- ICAEW Business Strategy and Technology Assessment Answer
- Unit 47: Business Intelligence Assignment Sample-BTEC-HND-Level 4 & 5
- Unit 47-LO2 Compare the tools and technologies associated with business intelligence functionality-BTEC-HND-Level 4 & 5
- Unit 47-LO3 Demonstrate the use of business intelligence tools and technologies-BTEC-HND-Level 4 & 5
- Unit 47-LO4 Discuss the impact of business intelligence tools and technologies for effective decision-making purposes and the legal/regulatory context in which they are used-BTEC-HND-Level 4 & 5
- Unit 43: Tapping into New and International Markets assignment sample-BTEC-HND-Level 4 & 5
- British Airways Marketing Case Study
- Apple Business Strategy Essay Sample
- Sainsbury’s Business Strategy Essay Sample
- Impact of Global Factors on UK Business Organizations Essay Sample
07 34272 Business Analytics Assignment Answer UK
07 34272 Business Analytics course is a dynamic and data-driven business landscape, the ability to make informed decisions based on accurate and timely information is crucial for organizations to gain a competitive edge. This course is designed to equip you with the knowledge and skills needed to harness the power of data and analytics to drive strategic decision-making and business performance.
Throughout this course, you will delve into the world of business analytics, exploring various concepts, techniques, and tools that enable you to extract valuable insights from vast amounts of data. You will learn how to collect, clean, analyze, and interpret data to identify patterns, trends, and correlations, and how to use these insights to make data-driven recommendations and optimize business processes.
Buy Non Plagiarized & Properly Structured Assignment Solution
Buy reliable assignments for 07 34272 Business Analytics course at the low cost!
At Students Assignment Help UK, we understand the significance of excelling in your 07 34272 Business Analytics course. That’s why we’re thrilled to offer you a remarkable opportunity to buy reliable assignments at an incredibly low cost. Our team of experienced writers, well-versed in the field of business analytics, is dedicated to crafting top-notch assignments tailored to meet your specific requirements. Our commitment to delivering high-quality work at affordable prices ensures that you receive exceptional value for your money.
In this section, we will discuss some assignment outlines. These are:
Assignment Outline 1: Critically evaluate the potential of analytic techniques in addressing business issues.
Analytic techniques have emerged as powerful tools for addressing business issues in the modern data-driven era. These techniques encompass a wide range of methods and technologies, such as statistical analysis, data mining, predictive modeling, and machine learning. When applied effectively, they can provide valuable insights, aid decision-making processes, and drive business growth. However, their potential is not without limitations and challenges. Let’s evaluate their potential critically.
- Data-driven decision making: Analytic techniques enable businesses to harness the power of data and make informed decisions. By analyzing large volumes of structured and unstructured data, organizations can identify patterns, trends, and correlations, leading to actionable insights. This can help optimize operations, improve efficiency, and identify new business opportunities.
- Predictive analytics: These techniques allow businesses to forecast future outcomes based on historical data. Predictive models help in demand forecasting, risk assessment, fraud detection, and customer behavior prediction. By leveraging these insights, organizations can proactively address potential issues, optimize resource allocation, and enhance strategic planning.
- Optimization and efficiency: Analytic techniques can optimize complex business processes, such as supply chain management, inventory management, and resource allocation. By applying mathematical optimization algorithms, businesses can streamline operations, reduce costs, and improve overall efficiency.
- Customer insights and personalization: Analytic techniques play a crucial role in understanding customer behavior and preferences. By analyzing customer data, businesses can segment their customer base, personalize marketing campaigns, and deliver targeted offerings. This leads to improved customer satisfaction, loyalty, and ultimately, increased revenue.
- Risk management and fraud detection: Analytic techniques help identify potential risks and detect fraudulent activities. Through anomaly detection algorithms and pattern recognition, businesses can mitigate financial risks, prevent fraud, and protect sensitive data.
Despite their potential, analytic techniques also face certain challenges and limitations:
- Data quality and availability: The effectiveness of analytic techniques heavily relies on the quality and availability of data. Inaccurate, incomplete, or biased data can lead to erroneous insights and flawed decision-making. Organizations must invest in data governance, data quality assurance, and data integration to overcome these challenges.
- Privacy and ethical considerations: Analytic techniques often involve the processing of personal or sensitive data. Organizations must adhere to privacy regulations and ethical guidelines to ensure data protection and maintain customer trust. Striking the right balance between data utilization and privacy can be a complex task.
- Skill and resource requirements: Analytic techniques require skilled professionals who possess a strong understanding of data analysis, statistics, and machine learning. Organizations may face challenges in hiring and retaining such talent. Additionally, implementing these techniques necessitates adequate technological infrastructure and computational resources, which can be costly.
- Interpretability and explainability: Some analytic techniques, such as complex machine learning models, lack interpretability. This poses challenges in explaining the rationale behind decisions to stakeholders and may hinder adoption in certain domains where interpretability is crucial, such as healthcare and finance.
- Domain knowledge and context: Analytic techniques alone cannot fully address business issues without domain knowledge and context. Understanding the specific business environment, industry dynamics, and market trends is essential for accurate interpretation of analytic results and their effective application.
Assignment Outline 2: Discuss ethical and privacy issues as they relate to analytics, machine learning and AI.
Ethical and privacy issues are of paramount importance when it comes to analytics, machine learning, and AI. Here are some key considerations related to these areas:
- Data Privacy: The use of analytics, machine learning, and AI often requires access to large amounts of data, including personal and sensitive information. Protecting individuals’ privacy and ensuring proper data handling practices are crucial. Organizations must obtain informed consent, anonymize data where possible, and implement robust security measures to prevent unauthorized access.
- Bias and Fairness: Analytics and AI systems can perpetuate existing biases present in the data they are trained on. Biased data can result in discriminatory outcomes and reinforce social inequalities. It is essential to identify and address bias during the development and deployment of these technologies to ensure fair and unbiased results for all individuals.
- Transparency and Explainability: Many machine learning and AI models are complex, making it challenging to understand their decision-making processes. Lack of transparency and explainability raises concerns about accountability and the potential for biased or unethical decision-making. Efforts should be made to develop interpretable models and provide explanations for the decisions made by these systems.
- Informed Consent and User Control: Individuals should have control over how their data is collected, used, and shared. Organizations must provide clear information and obtain informed consent from users regarding the data collection and processing activities performed by analytics and AI systems. Users should have the ability to modify their preferences and opt-out if desired.
- Impact on Employment: The increasing adoption of analytics, machine learning, and AI can lead to automation and displacement of certain jobs. Organizations and policymakers need to consider the potential societal and economic impacts, including retraining and upskilling programs, to ensure a smooth transition for affected individuals.
- Accountability and Liability: As analytics and AI systems become more autonomous, questions arise regarding who should be held accountable for their actions and any potential harm caused. Clear frameworks and regulations are needed to determine liability and ensure that developers, users, and organizations using these technologies are held responsible for any negative consequences.
- Dual-Use Dilemma: Analytics, machine learning, and AI technologies can have both beneficial and harmful applications. While they can be used for improving healthcare, education, and public services, they can also be misused for surveillance, propaganda, or other malicious purposes. Ethical considerations must be at the forefront to prevent misuse and ensure the responsible development and deployment of these technologies.
To address these issues, organizations and policymakers should proactively develop and adhere to ethical guidelines, foster interdisciplinary collaborations, promote diversity and inclusivity in development teams, and engage in ongoing public dialogue about the implications of analytics, machine learning, and AI on society.
Assignment Outline 3: Critically evaluate the role of big data in business.
Big data plays a significant role in today’s business landscape, offering numerous opportunities and challenges for organizations across various sectors. While big data holds immense potential for businesses, it is crucial to evaluate its role critically. Here are some key points to consider:
- Decision-Making: Big data provides organizations with vast amounts of information that can aid in decision-making. By analyzing large datasets, companies can gain valuable insights into consumer behavior, market trends, and operational efficiency. These insights enable data-driven decision-making, helping businesses identify new opportunities, optimize processes, and improve overall performance.
- Competitive Advantage: Organizations that effectively leverage big data can gain a competitive edge. By harnessing advanced analytics and machine learning techniques, companies can uncover patterns, correlations, and trends that were previously inaccessible. This allows them to develop innovative products, personalize marketing strategies, enhance customer experiences, and make strategic business moves.
- Customer Insights: Big data enables businesses to gain a deeper understanding of their customers. By analyzing customer data, including demographics, preferences, and purchasing behavior, companies can create targeted marketing campaigns and tailored product offerings. This enhances customer satisfaction, increases loyalty, and improves customer retention rates.
- Operational Efficiency: Big data analytics can help optimize internal processes and enhance operational efficiency. By analyzing large volumes of data, companies can identify bottlenecks, inefficiencies, and areas for improvement. This information enables businesses to streamline operations, reduce costs, and make data-driven decisions to enhance productivity.
- Risk Management: Big data can assist organizations in identifying and managing risks more effectively. By analyzing vast datasets, businesses can detect potential fraud, predict market fluctuations, and identify patterns that indicate operational or financial risks. This enables proactive risk mitigation strategies and improves overall risk management practices.
- Ethical and Privacy Concerns: The use of big data raises ethical and privacy concerns. Collecting and analyzing massive amounts of data can intrude on individuals’ privacy, leading to potential misuse of personal information. Businesses must prioritize data security, compliance with privacy regulations, and transparent data practices to address these concerns.
- Data Quality and Interpretation: The quality and accuracy of data used for analysis are crucial. Inaccurate or incomplete data can lead to incorrect conclusions and misguided decisions. It is essential for organizations to invest in data governance frameworks, data validation processes, and robust data analytics capabilities to ensure data accuracy and interpret findings correctly.
- Scalability and Infrastructure: Big data requires significant computational power, storage capabilities, and infrastructure investments. Organizations need to have the necessary resources to collect, store, process, and analyze large datasets. Implementing scalable systems and cloud-based solutions can help address these challenges and enable efficient data management.
Please Write Fresh Non Plagiarized Assignment on this Topic
Assignment Outline 4: Select, evaluate and apply data extraction techniques to real-world datasets.
When it comes to data extraction techniques for real-world datasets, there are several approaches you can consider. The selection of a technique depends on the nature of the dataset and the specific requirements of your analysis. Here are a few commonly used data extraction techniques along with their evaluation and application:
Web Scraping:
- Technique: Web scraping involves extracting data from websites by parsing the HTML structure of web pages.
- Evaluation: Assess the complexity of the website’s HTML structure, availability of data, and legality of scraping.
- Application: Extracting product information, social media data, news articles, or any publicly available data on the web.
APIs (Application Programming Interfaces):
- Technique: APIs allow direct access to data provided by various platforms and services.
- Evaluation: Evaluate the availability and quality of APIs, authentication requirements, and rate limits.
- Application: Extracting data from social media platforms, weather data, financial data, and other online services.
Database Queries:
- Technique: Execute SQL queries or use specific query languages to retrieve data from structured databases.
- Evaluation: Understand the database schema, data structure, and query performance.
- Application: Extracting data from relational databases like MySQL, PostgreSQL, or Microsoft SQL Server.
File Parsing:
- Technique: Parse structured files, such as CSV, JSON, XML, or log files, to extract relevant data.
- Evaluation: Consider the file format, schema complexity, and the need for custom parsers.
- Application: Extracting data from flat files, log files, or structured data files generated by different software.
Natural Language Processing (NLP):
- Technique: Utilize NLP techniques to extract structured data from unstructured text documents.
- Evaluation: Evaluate the accuracy of NLP models for text extraction and entity recognition.
- Application: Extracting information from articles, research papers, customer feedback, or any textual data.
Optical Character Recognition (OCR):
- Technique: OCR techniques extract text from images or scanned documents.
- Evaluation: Assess OCR accuracy, especially for complex document layouts or low-quality images.
- Application: Extracting data from scanned documents, invoices, receipts, or any text embedded in images.
While selecting and applying data extraction techniques, ensure that you comply with legal and ethical considerations, such as respecting website terms of service, privacy policies, and data usage rights. Additionally, consider the scalability, maintainability, and automation aspects of the chosen techniques based on the volume and frequency of data extraction required.
Assignment Outline 5: Critically appraise techniques used for data cleaning, manipulation, and analysis and apply these techniques to real-world datasets using industry standard software tools.
Data cleaning, manipulation, and analysis are crucial steps in the data science and analytics pipeline. They involve preparing raw data for further analysis by addressing issues such as missing values, outliers, inconsistencies, and transforming data into a suitable format. Various techniques and industry-standard software tools can be used for these tasks. Here, I’ll provide an overview of common techniques and tools for data cleaning, manipulation, and analysis.
- Data Cleaning Techniques:
- Handling missing values: Techniques such as deletion (listwise or pairwise), imputation (mean, median, regression imputation), or advanced methods like multiple imputation can be used to handle missing values.
- Outlier detection: Statistical methods like z-score, modified z-score, or distance-based approaches (e.g., clustering-based outlier detection) can be used to identify and handle outliers.
- Data standardization: Standardizing numerical data (e.g., z-score normalization) can be performed to bring different variables to a common scale.
- Handling inconsistencies: Techniques like data profiling, domain knowledge-based validation, and rule-based checks can be used to identify and address inconsistencies in the data.
- Data Manipulation Techniques:
- Data merging and joining: Combining multiple datasets based on common variables or using different types of joins (inner join, outer join, etc.) can be done to consolidate data from different sources.
- Data transformation: Techniques like scaling, log transformation, feature engineering, and creating derived variables can be applied to transform data for better analysis.
- Aggregation and summarization: Summarizing data using techniques such as grouping, pivoting, and aggregation functions can provide insights into patterns and trends.
- Splitting and sampling: Dividing data into subsets or sampling techniques (e.g., random sampling, stratified sampling) can be employed for various purposes, such as model validation or reducing computational burden.
- Data Analysis Techniques:
- Descriptive statistics: Calculating measures such as mean, median, standard deviation, or generating frequency distributions can provide initial insights into the dataset.
- Exploratory data analysis (EDA): Techniques like data visualization (plots, charts, graphs) and summary statistics can be used to explore relationships, identify patterns, and gain a deeper understanding of the data.
- Statistical modeling: Employing statistical techniques such as regression analysis, hypothesis testing, ANOVA, or machine learning algorithms for predictive modeling and inference.
- Text mining and natural language processing (NLP): Analyzing unstructured text data using techniques like sentiment analysis, topic modeling, or named entity recognition.
- Industry Standard Software Tools:
- Python: Libraries like Pandas, NumPy, and SciPy provide extensive functionalities for data cleaning, manipulation, and analysis. Jupyter Notebook and Anaconda are widely used for interactive data analysis.
- R: R programming language and packages like dplyr, tidyr, and ggplot2 offer powerful tools for data manipulation and visualization.
- SQL: Structured Query Language (SQL) is commonly used for data manipulation tasks, especially in working with relational databases.
- Excel: Microsoft Excel provides basic data cleaning and manipulation features, making it accessible for simple data tasks.
- Tableau, Power BI: These tools are popular for data visualization and exploratory analysis, allowing interactive exploration of data.
By combining appropriate techniques and utilizing industry-standard software tools, data scientists and analysts can effectively clean, manipulate, and analyze real-world datasets, leading to valuable insights and informed decision-making.
Assignment Outline 6: Select and implement techniques for data visualisation and apply this to real-world datasets.
When it comes to data visualization, there are numerous techniques and tools available to present data effectively. I’ll provide an overview of some popular techniques and their applications, and then demonstrate how to apply them using real-world datasets. Let’s get started!
- Line Charts: Line charts are ideal for displaying trends and patterns over time. They are useful for visualizing stock prices, population growth, or any data with a time component.
- Bar Charts: Bar charts are excellent for comparing categorical data. They are commonly used to display survey results, market shares, or any data with distinct categories.
- Pie Charts: Pie charts are suitable for displaying proportions or percentages. They work well for illustrating market segments, budget allocations, or any data where you want to show the composition of a whole.
- Scatter Plots: Scatter plots are ideal for visualizing the relationship between two continuous variables. They are commonly used in scientific research to show correlations or clusters in data.
- Heat Maps: Heat maps use color-coding to represent data values across a matrix. They are helpful for displaying geographic data, indicating density, or showing patterns in large datasets.
- Geographic Maps: Geographic maps are powerful for visualizing data with a spatial component. They are commonly used to display demographic data, weather patterns, or any information tied to specific locations.
- Treemaps: Treemaps are effective for visualizing hierarchical data. They use nested rectangles to represent proportions and sizes of different categories within a hierarchy.
Now, let’s apply these techniques to real-world datasets using Python and the matplotlib library.
Pay & Get Instant Solution of this Assignment of Essay by UK Writers
Obtain Genuine Assignment Solutions for 07 34272 Business Analytics at a Reasonable Cost with Zero Plagiarism!
The assignment sample mentioned above is specifically related to the subject of Business Analytics, with the course code 07 34272. It serves as a demonstration of the high-quality work delivered by our team of business assignment experts at Students Assignment Help UK. We take pride in providing exceptional assistance to students in various academic disciplines.
Aside from business assignments, we also offer a cheap essay writing service in the UK. Our professional writers are well-versed in diverse subjects and can produce top-notch essays that meet your requirements. Furthermore, if you are struggling with your homework and need guidance, you can hire homework helper from us. Our dedicated team of experts is equipped with extensive knowledge in their respective fields and can provide comprehensive assistance with your homework tasks.
At Students Assignment Help UK, we aim to provide reliable and efficient assignment help online UK. We understand the challenges students face in their academic journey, and our services are tailored to alleviate their stress and enhance their learning experience.
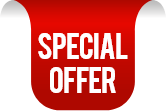
UPTO35%
Avail The Benefit Today!
To View this & another 50000+ free
do you want plagiarism free & researched assignment solution!
UPTO 35% DISCOUNT
Get Your Assignment Completed At Lower Prices