- NVQ Level 5 Unit 507 Safeguarding in Adult Care
- Structural Design of a 4m Roof Beam: Load and Stability Analysis, Assessment 2
- M33118 Configuring and Studying ASA Basic Settings and Firewall Using CLI
- The Reasons For Hitler Gaining Power In 1933, Course Work
- Sensitivity Analysis of Option Pricing: Impact of Volatility and Barrier Conditions
- Network Threats and Vulnerabilities, UNIS, Coursework
- Corporate Finance and Business Valuation Coursework
- 5301ELE Digital System Evaluation Coursework, Level 5, LJMU 2024
- Power Electronic Applications and Control Coursework 1 2024
- PE7007 Construction Economics, Coursework Brief – 2024/2025 , NU, UK
- Level 3 Diploma in Adult Care, Coursework, UK: Understand the application of personcentred practices in care settings
- Decide whether each of the following statements is true or false. Justify your answer in each case by giving an example or stating any general result seen at the lecture: Mathematics, Coursework, NU, UK
- 7CO03: Critically assess different ethical standpoints on people practice and the maintenance of high standards of ethical behaviour: CIPD level 7 , Coursework, UK
- SQE2: Oral Skills-Written Skills- Apply the law comprehensively to the client’s situation, identifying any ethical and professional conduct issues: Reflective Portfolio, Coursework, UK
- SQE 1 Preparation: Portfolio Assessment-analyse and reflect upon your development of the practical legal skills that the Solicitors Regulation Authority (the SRA) : Reflective Portfolio, Coursework, UK
- DSM120: Financial Data Modelling, Coursework 1, UK
- DSM070: Building the Blockchain: the chain, mining, and the consensus mechanism: Blockchain Programming, Coursework 3, UOL, UK
- FHEQ Level 7 ES5800 – Systematic review protocol In this assessment you are asked to write a systematic review protocol: Analytical skills for Environmental Managers, Coursework, BUL, UK
- FHEQ Level 7 ES5800 – Data analysis As part of your learning activities in the autumn 2023 term, you have measured reaction times using the ruler drop test among athletes: Analytical skills for Environmental Managers, Coursework, BUL, UK
- FHEQ Level 7 ES5800 – Analytical skills for Environmental Managers, Coursework, BUL, UK
AM41PB: Bayesian approach – The Pareto distribution is a power-law-like probability distribution that describes the distribution of wealth in a society: Probabilistic Modelling Coursework, UOM, UK
University | University of Milan (UOM) |
Subject | AM41PB: Probabilistic Modelling |
- Bayesian approach – The Pareto distribution is a power-law-like probability distribution that describes the distribution of wealth in a society, fitting the trend that a large portion of wealth is held by a small fraction of the population. It takes the form
where it provides the probability of x above a threshold Xm ≥ 0
On BlackBoard, under the section assignments, please find a dataset income distribution by percentile of the UK population for the tax years 2010-11 to 2018-9. Use the last digit of your student number to choose the tax year you will be working on (0 → 2010 − 11, 1 → 2011 − 2, . . . , 8, 9 → 2018 − 9).
- Show that the Pareto distribution is indeed a probability distribution.
- Plot the data as the percentage of the population (y-axis) with income above certain values (x-axis); to simplify the presentation present the income in thousands of pounds.
- Assuming the data is i.i.d. and represented by the Pareto distribution, find the maximum likelihood expression for the parameter λML. Obtain the numerical value of λML given data D and Xm = £15, 000. Specify the data you are working with and the number of data points used.
- For data set D = {x1, . . . , xN }, show that the Gamma probability distribution function
is the conjugate prior for the Pareto distribution? Derive the corresponding posterior p(λ|D). - Assuming the data is represented by the Pareto distribution, find the Maximum A Posteriori expression of the parameter λMAP. Use the prior values α = 1 and β = 1 to obtain the numerical value of λMAP given data D.
- Use the values obtained for the parameters λML and λMAP to compare the estimated percentage of the population with income above £20, 000.
- Decision theory- Large Diamonds (D) are exponentially rare and their weight follows the exponential distribution
where g is the weight of a diamond in grams and λD is a constant. In the same mine, there are also White Sapphire (WS) crystals that look like diamonds but are very regular in weight, following a Gaussian distribution.
of mean µS and variance σ, 2 S. The average size of diamonds λD is much smaller than that of White Sapphire λD << µS. Additionally, diamonds are much rarer, so p(WS) << p(D).
The mine owner wants to automate the sorting of Diamonds vs White Sapphire by weight, setting a threshold weight gT.
- Write an expression for the misclassification error of Diamonds as White Sapphire p(D|WS) and of White Sapphire as Diamonds p(WS|D) as functions of the threshold weight gT.
- Minimize the total misclassification error with respect to the threshold weight gT. What is the expression obtained for gT.
- Calculate the value of gT for the parameters λD = 1, µS = 5, σ2 S = 1 and prior probabilities p(WS) = 0.8 and p(D) = 0.2. Sketch the two distributions, point to the threshold value gT and to the area that contributes to the misclassification error of Diamonds as White Sapphire.
- The mine is losing money and the owner realizes that he has not taken into account that large diamonds are exponentially more expensive and the misclassification cost p(WS|D) with respect to p(D|WS) (which for simplicity we will attribute cost 1 to) is
Assume λC < λD. Find the threshold weight gT expression in this case (not numerical value).
Buy Answer of This Assessment & Raise Your Grades
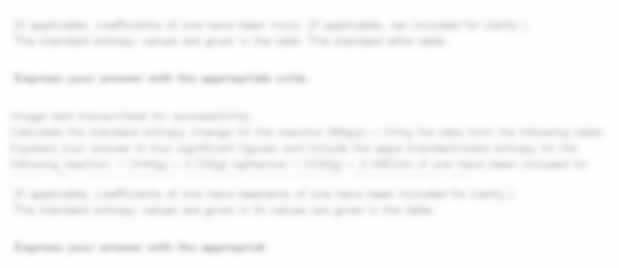