- NVQ Level 5 Unit 507 Safeguarding in Adult Care
- Structural Design of a 4m Roof Beam: Load and Stability Analysis, Assessment 2
- M33118 Configuring and Studying ASA Basic Settings and Firewall Using CLI
- The Reasons For Hitler Gaining Power In 1933, Course Work
- Sensitivity Analysis of Option Pricing: Impact of Volatility and Barrier Conditions
- Network Threats and Vulnerabilities, UNIS, Coursework
- Corporate Finance and Business Valuation Coursework
- 5301ELE Digital System Evaluation Coursework, Level 5, LJMU 2024
- Power Electronic Applications and Control Coursework 1 2024
- PE7007 Construction Economics, Coursework Brief – 2024/2025 , NU, UK
- Level 3 Diploma in Adult Care, Coursework, UK: Understand the application of personcentred practices in care settings
- Decide whether each of the following statements is true or false. Justify your answer in each case by giving an example or stating any general result seen at the lecture: Mathematics, Coursework, NU, UK
- 7CO03: Critically assess different ethical standpoints on people practice and the maintenance of high standards of ethical behaviour: CIPD level 7 , Coursework, UK
- SQE2: Oral Skills-Written Skills- Apply the law comprehensively to the client’s situation, identifying any ethical and professional conduct issues: Reflective Portfolio, Coursework, UK
- SQE 1 Preparation: Portfolio Assessment-analyse and reflect upon your development of the practical legal skills that the Solicitors Regulation Authority (the SRA) : Reflective Portfolio, Coursework, UK
- DSM120: Financial Data Modelling, Coursework 1, UK
- DSM070: Building the Blockchain: the chain, mining, and the consensus mechanism: Blockchain Programming, Coursework 3, UOL, UK
- FHEQ Level 7 ES5800 – Systematic review protocol In this assessment you are asked to write a systematic review protocol: Analytical skills for Environmental Managers, Coursework, BUL, UK
- FHEQ Level 7 ES5800 – Data analysis As part of your learning activities in the autumn 2023 term, you have measured reaction times using the ruler drop test among athletes: Analytical skills for Environmental Managers, Coursework, BUL, UK
- FHEQ Level 7 ES5800 – Analytical skills for Environmental Managers, Coursework, BUL, UK
IMAT3613: Data Mining Report A Patchwork Assignment. analyze the Organics dataset within SAS Enterprise Miner or Weka: Data Mining Course Work, DMU, UK
University | De Montfort University (DMU) |
Subject | IMAT3613: Data Mining |
Learning Outcomes
- To discuss what is meant by the term data mining, to be able to express a business problem within a data mining framework and identify an appropriate target variable.
- To be able to identify necessary prerequisites for the application of a data mining framework and discuss the limitations of data mining methods with regard to data and methods in the context of the business problem.
- To describe and analyze the organizational structure of large data sets to facilitate effective data mining and to be able to correctly interpret and critically evaluate the results to make informed decisions within a data mining framework
- To express the data mining framework for a particular business problem through the correct application of data mining tools.
- To interpret the results produced from data mining tools and evaluate the effectiveness of data mining methods and where necessary make appropriate recommendations for use in the virtuous cycle of data mining.
Data Mining Report A Patchwork Assignment
Structure of the coursework.
The coursework is an individual piece of assessment, requiring you to analyze the ORGANICS dataset within SAS Enterprise Miner or Weka, using the directed data mining techniques covered in the IMAT3613 module, and detailing your results, and interpretations, conclusions, and recommendations in a well-structured technical report. You are provided with:
- This Brief
- A sample of data from the ORGANICS dataset is shown in Appendix A.
- The ORGANICS dataset contains 10,000 observations and 13 variables. The variables in the dataset are shown in Appendix B.
- The coursework will be assessed according to the marking grid in Appendix C
- Self/Peer Assessment Rubric Appendix D
- Template Report in Appendix E.
Do You Need Assignment of This Question
Lab Journal and Reflection
To help your produce this report in a timely manner, the report is built up from four biweekly activities. You have an opportunity to modify your work from each activity in light of your own reflection and self-assessment feedback. Ten percent of the marks are awarded for a reflection on how you have developed your report over the term. The length of this reflection should be at least 200 words and is to be included in your appendix. To help you produce this reflection you may make use of the journal feature on the blackboard and the self-assessment grid to record your progress.
The last and fifth activity is to produce an integrated report with conclusions and recommendations you will complete independently.
In the odd weeks, it is suggested that you upload your answer to the activity on the lab journal.
In the even weeks, you are expected to comment on your work using the self-assessment rubric and assign a grade of A, B, C, D, or F. At the beginning of the even weeks, a rubric will be produced for each activity to guide you in the assessment of your self-assessment. These marks are not used to make up the final mark however your engagement with the process is. This has been designed to help you structure your work and pace the development of the report over the term.
You may modify your weekly contributions through your engagement in the lab journal. In fact, you are encouraged to do so. You should treat the lab journal as a notebook of your activities for the week.
In the final exercise, you will integrate all four activities and the final activity into a report.
In each activity you are expected to produce a piece of writing from between 200 and 400 words, producing a final report to a maximum of 2000 words excluding, a table of contents, diagrams, and appendices. You are provided a template report to complete, existing words in the template do not count to the reported maximum.
This type of assessment is known as a patchwork assessment and is more UDL-friendly compared to traditional forms of report assessment.
The patchwork assessment gives you an opportunity to improve your work over the term and reduces the stress of having to produce one piece of writing at the last minute.
The final report is summative and is marked by your tutor according to the attached marking grid.
Individual Data Set
You will each individually generate a unique model set personal to you.
Each of you will be working on your own random sample of data generated by typically inserting the last 5 figures of your DMU student id number into the random seed generator within the Data Partition node. You will be shown how to do this in the labs.
Note: If the spurious output for any of the models should occur, insert the last 4 figures (or the last 3 figures) of your DMU student id number into the random seed generator – to enable you to generate sensible output that you can interpret.
In SAS Enterprise Miner
In Weka
If you are using Weka, you should randomize your data set before producing the training and test set using a randomizer filter. This can be found in filters> unsupervised>instances>randomizer
Submission
You will need to submit a copy of your report using the Turnitin link in the assessments section of the Data Mining module shell on Blackboard (to be made available prior to the coursework deadline).
Provision of Data mining tools and notes on using Weka
You may use either SAS Enterprise Miner or Weka as your platform to generate models it does not matter which. Weka is the alternative software option that is recommended if you have problems with accessing campus computers or you have been unable to install SAS enterprise miner on your home PC. This is likely to appeal to MacOS users who are not comfortable with setting up and using virtual machines or do not have the hardware resources to use Enterprise Miner. Weka is an open-source software from the University of Waikato.
The learning resources presented in the module schedule use SAS Enterprise Miner, and have more integrated support by way of screencast videos. If for some reason you wish to use Weka, please use the resources listed in the “using Weka” handout, note these resources are largely self-directed.
You are provided with an AIFF file for the HMEQ data set and some guidance on how you can adapt the HMEQ lab activities to using the WEKA platform. It is recommended that you use SAS enterprise miner if at all possible. Due to the effort required to learn a tool you should fix the platform you are going to use within the first two weeks. This provision is only made to those students who are facing technical difficulties with their hardware.
Note nearly everything you can do in SAS enterprise miner you may be able to do in Weka with the exception of the following functionality:
There is no means to produce non-cumulative lift charts; use cumulative lift chart, AUC, TPR and classification measures as percentages instead. Note unlike SAS you cannot compare different sized datasets in the Model performance chart, use percentages were possible when comparing
- different charts so you can make a direct comparison. Do not report frequencies but present percentages when presenting data for true positives, true negatives, false positives, and false negatives. Note that Weka uses the term sample size for depth in its generation of a lift chart, the range goes from 0 to1, which is equivalent to 0 to 100%
- The use of the test set is not integrated into Weka. You must split your data into three, training, validation, and test set. Recombine training and validation, this then becomes the input for Weka for model building. Note in Weka’s language this is called train test which is the equivalent of train validation in SAS enterprise miner. Separately you will need to create a test set that should correspond to 30% of the original data and treat this as a hold-out set. When you have selected your champion model use this to assess model performance on unseen data. You will need to use the serialized instance saver to export the model and serialisedinstanceloader nodes to score the test data. This is unnecessary in SAS enterprise miner as the test set performance is assessed directly in the model comparison node.
- The modify functionality is best used in the pre-process tab of Weka’s explorer do not use the knowledge flow to do pre-processing. Create separate model sets (Train/Test) missing values, treatment of outliers, and transformations as separate arff files. This is suggested in the guidance notes. SAS takes care of this directly, Weka does not so you will have to apply these transformations to the “hold out” set before you score it.
d)p-values for logistic regression coefficients are not presented in Weka, the advice is to use the information gain and rank on the attribute selection function in Weka, or J48 attribute selection for variable selection in logistic regression. Note the default behaviour for logistic regression node in Weka is that it imputes the mean and the mode of missing values. If you want to assess model performance on missing values you must create a separate arff file and remove these values using the filters. See guidance notes.
Buy Answer of This Assessment & Raise Your Grades
Are you concerned about finishing your IMAT3613: Data Mining coursework task? then you are at the right place. we, at Students Assignment Help UK presents outstanding coursework writing help at a low price. They know all kinds of formats. like APA, MLA, Harvard, Chicago, Oxford, and others as per the university guidelines.
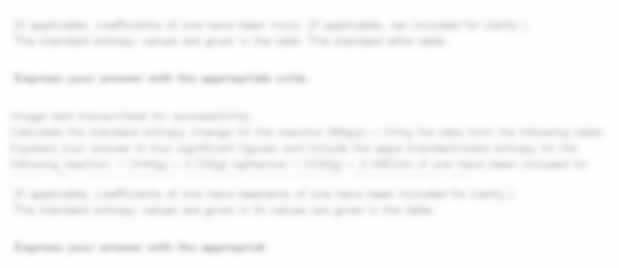